Customer challenge: Lack of a unified way-of-working among data science teams, resulting in a longer lead-time to production for AI solutions. Difficulty hiring the right profiles for data engineering and setting up the correct platform architecture.
Provided solution:
AI MATURITY SCAN assessing Randstad’s data and capabilities, followed by strong support by one of our lead data scientists and a lead data engineer. Xebia consultants introduced standardized ways-of-working, set up chapters, supported hiring, and improved knowledge of data teams.
Outcome: Randstad’s data scientists now form one team that works more effectively and efficiently drastically reducing the lead time to production for AI solutions. With the help of Xebia’s lead data engineer, this data engineering team has expanded and improved its way-of-working.
Dutch multinational human resource consulting firm Randstad had a problem. Actually, it had a few of them. Its multiple data science teams lacked a unified way of working, so the lead-time on producing AI solutions was too long. On top of that, the company was having trouble finding the right people to hire to do its data engineering. It also wasn’t setting up the correct platform architecture. Randstad needed a more than a “quick-fix” solution it wanted to develop a First Class Data Science and Engineer practice.
Bringing the Tech to Randstad’s Touch
Improving the company’s data science practice was one of the goals Vermeer set out to achieve when he joined Randstad as a data and analytics manager in late 2016. “Most people think of HR as a ‘people process,’ so when I joined, not much was informed or driven by data,” he explains. He convinced the company to implement a “data driven” approach so that decisions were based on facts & data instead of gut feeling.
“I spent about 20% to 30% of my time educating business users at Randstad and was often met with surprise. As an HR company, not many people are aware of the possibilities of data, and most employers don’t know about the possibilities data offers,” he explains.
Despite his efforts to educate the company on the benefits of data analysis, he encountered lots of challenges. He explains, “People get used to making decisions based on feelings, so changing that mindset took some time, naturally.”
Looking For a Standard Way-of-Working
After running several successful data science pilots and use case, Vermeer and his team ran into two issues:
- Industrializing models didn’t go smoothly
- The data scientists lacked a common way-of-working
“The tools weren’t the problem; the challenge was in using them in a reproducible way,” he says. “The issue was further complicated by the different ways-of-working and different visions among the data scientists.” So Vermeer set out to find external help to solve these issues.
“We spoke with several parties and not only did Xebia stand out in expertise, but it also showed an ability to make a real connection with us. That human side was exactly what we were looking for,” he says.
AI Maturity Scan as a Starting Point
Xebia executed its AI MATURITY SCAN at Randstad to create a baseline of the organization’s AI capabilities that:
- Created a clear picture of the organization’s current capabilities
- Set a baseline against which it could mark historical and future progress
- Laid a solid foundation for the consulting work of Xebia
“For me, the outcome of the scan confirmed what I already experienced in everyday practice. But it was also valuable to have hard evidence to show the rest of the organization what we were up against,” Vermeer explains. “It also gave Steven Nooijen, the Xebia consultant who would eventually work with us, a clear picture of where we were at before he started the assignment.”
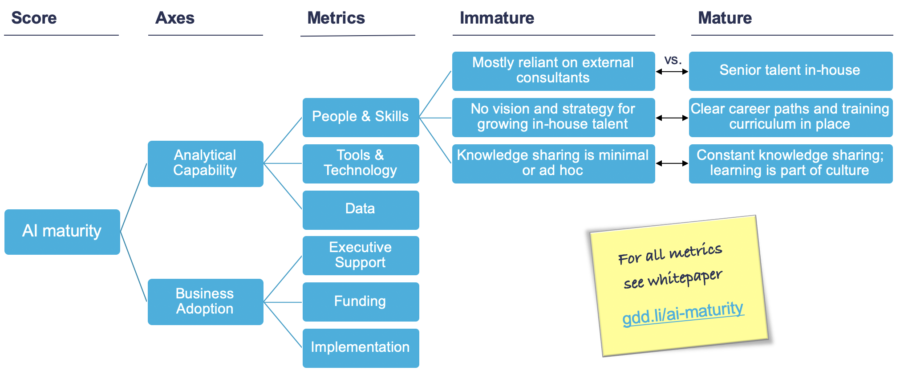
Figure 1: Driver tree showing how AI maturity can be measured by looking into immature and mature practice examples for each of the AI maturity metrics.
3 STEPS TO GROW YOUR ORGANIZATION’S AI COMPETENCY
Chapter Lead for Data Science Teams
Nooijen’s primary assignment was to bring together the data scientists working at Randstad and form a chapter. Vermeer paints the picture: “When Steven started, there was no unified way-of-working. Our data scientists came from many different backgrounds—some used R, some used Python—they were spread across multiple teams, making the challenge even bigger. We had tried to create standards before, but hadn’t succeeded.”
After a year of working with the team at Randstad, Vermeer sees distinct differences and improvements: “The data scientists are now a team; they work better together, and communication is better. It’s all been very successful.” He also applauds Nooijen’s approach, “Steven doesn’t barge in and tell people how they should work; instead, he leads and encourages the entire data science team in collaboration, while being part of it.”
While at Randstad, Nooijen also mapped a growth path for the data scientists there, with an overview of the expertise required for junior, mid-level, and senior data scientists. He linked this map to a training plan that indicated the correct courses for each level. “It has already helped the team advance its capabilities,” smiles Vermeer.
At the end of his assignment at Randstad, Nooijen helped the company find and hire a full-time, internal lead data scientist. Vermeer smiles, “We can’t ever truly replace Steven, but we’re confident the person we’ve hired will also do an exceptional job. He’s excited about the opportunity, and that is in large part, thanks to the work Steven has done together with the data science team.”
Setting a Data Engineering Standard
Six months after bringing in Steven Nooijen as the lead data scientist, Randstad hired Tünde Alkemade, a lead data engineer from Xebia. Vermeer explains his team’s decision: “We needed to create a technical benchmark for our AWS platform and speed up the professionalization of our data engineering department. Tünde’s experience with AWS at previous assignments proved incredibly valuable in this assignment. We also needed the right data engineers to work on the platform, but struggled to find the right profiles. We had applicants, but to assess their technical skills and fit with our technology is where Tünde also proved her worth.”
Tünde’s will continue at Randstad and help the company form a chapter of data engineers.
Reflecting on the collaboration with Xebia, Vermeer is impressed:
“When I see how fast we can deploy a model now, and also our increased flexibility, I’m amazed by the vast difference compared to five months ago. It’s also great that Steven and Tünde did this together with our teams. The connection between data engineering and data science has improved and there is now a data science community within the company. I couldn’t be more pleased.”
About Randstad
Randstad is the second-largest staffing company in the world, active in 33 countries, with over 4,861 offices. Randstad employs more than 38,000 people, and every day over 649,000 candidates work through Randstad. In 2019, Randstad’s revenue stood at €23.7 billion, with a net profit of €766 million.
Industry
AI MaturityHR & Recruitment
Organize
Project type
AI Maturity ScanSetting-Up Platform Architecture
Technologies used
Analytics Translator“When I see how fast we can deploy a model since working with Xebia, and also at the increased quality, I’m amazed by the vast difference compared to five months ago”
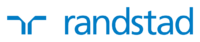